Analysis of New Zealand GREENGrid household electricity demand data conducted by Dr Ben Anderson and colleagues at the University of Otago as part of Ben’s MSCA Global Fellowship have provided unique insights into the compnents of peak residential electricity demand in New Zealand.
The analysis, which was also supported by the New Zealand Energy Efficiency and Conservation Authority (EECA), showed that although the data does not derive from a representative sample of households there is evidence that:
- identifiable heating contributes ~ 21% to residential peak (17:00-21:00) demand in winter
- Hot Water contributes ~ 17%
- Lighting 14%
- Ovens 7% and
- non-identified appliances contribute ~ 40%.
These percentage contributions are generally similar at both regional network and sample co-incident peaks and across seasons although total power demand varies by season according to the appliance. Thus ‘Others’, heating and lighting are substantially lower in summer and whilst further research is clearly needed to unpack ‘Other’ demand, this suggests heating may be a major component.
The work also drew on Ben’s Fellowship work on small area estimation to develop examples of upscaling the GREENGrid household sample to the regional and national level. This work showed that the GREEN grid data is insufficient for this purpose due to:
- its small size – meaning very small numbers of households would be ‘representing’ very large numbers in the true population with a consequential risk of both skew and a lack of precision;
- its recruitment bias – meaning that some household types and some crucial energy source types (e.g. coal, wood-only, reticulated gas) are not represented at all and so cannot be ‘re-weighted’.
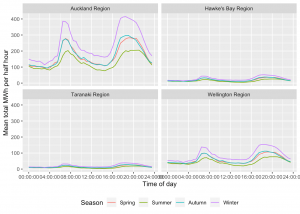
Estimated total residential electricity consumption per half-hour by season for selected regions (2015)
This was demonstrated through a number of worked examples and suggests that future work could apply these methods to more suitable larger scale data sources. However the work also showed that that small and biased samples such as GREENGrid can be used to create scenarios of future demand at national, regional or small area (neighbourhood) levels where the sample comprises a set of households specifically chosen to represent ‘the future’, re-weighting methods can be used to develop plausible estimates of their future demand profiles.
Where this is not the case, specific household attributes can be changed in specific households in the sample and the consequences upscaled to local, regional or national estimates – an approach known as spatial microsimulation.